Applied example: RTC time series analysis#
Now that we have done so much work to organize these two datasets and prepare them for analysis, let’s explore the data with a scientific question in mind.
In this example, we have data over a specific area of interest covering two glaciers and two proglacial lakes in the Central Himalaya near the India-Chinese border. As a glaciologist, I might be interested in questions related to the conditions of these surface – is there a seasonal pattern to proglacial lake conditions? Do they freeze during the winter, and if so, at similar times? What is the surface of the glacier like at certain times of year? SAR backscatter imagery may not conclusively answer any of these questions in itself, but it can provide important insights about surface conditions and how they change over time that could be used to answer some of these questions.
This notebook will walk through some initial steps of how you could use the data objects we’ve created to explore backscatter dynamics over time and space for the area of interest we have identified.
Learning objectives#
Concepts#
Subset larger dataset to spatial areas of interest
Computations and reductions
Data visualization
Techniques#
Other useful resources#
These are resources that contain additional examples and discussion of the content in this notebook and more.
How do I… this is very helpful!
Xarray High-level computational patterns discussion of concepts and associated code examples
Parallel computing with dask Xarray tutorial demonstrating wrapping of dask arrays
Software and setup#
%xmode minimal
Exception reporting mode: Minimal
import geopandas as gpd
import xarray as xr
from shapely import geometry
import matplotlib.pyplot as plt
import numpy as np
Utility functions#
def get_bbox_single(input_xr, buffer = 0):
'''Takes input xr object (from itslive data cube), plots a quick map of the footprint.
currently only working for granules in crs epsg 32645'''
xmin = input_xr.coords['x'].data.min()
xmax = input_xr.coords['x'].data.max()
ymin = input_xr.coords['y'].data.min()
ymax = input_xr.coords['y'].data.max()
pts_ls = [(xmin, ymin), (xmax, ymin),(xmax, ymax), (xmin, ymax), (xmin, ymin)]
crs = input_xr.rio.crs
polygon_geom = geometry.Polygon(pts_ls)
polygon = gpd.GeoDataFrame(index=[0], crs=crs, geometry=[polygon_geom])
polygon_prj = polygon
polygon = polygon_prj.to_crs(crs)
#add a buffer if needed
bounds = polygon.total_bounds
#bounds = [bounds[0]-500, bounds[2]+500, bounds[1]-500, bounds[3]+500]
bounds_xmin = bounds[0]-buffer
bounds_xmax = bounds[2]+buffer
bounds_ymin = bounds[1]-buffer
bounds_ymax = bounds[3]+buffer
bounds_ls = [(bounds_xmin, bounds_ymin), (bounds_xmax, bounds_ymin),
(bounds_xmax, bounds_ymax), (bounds_xmin, bounds_ymax),
(bounds_xmin, bounds_ymin)]
bounds_geom = geometry.Polygon(bounds_ls)
bound_gdf = gpd.GeoDataFrame(index=[0], crs=crs, geometry = [bounds_geom])
bounds_prj = bound_gdf.to_crs(crs)
return bounds_prj
def power_to_db(input_arr):
return (10*np.log10(np.abs(input_arr)))
Read in prepared RTC data#
this example will use ASF dataset
%store -r vrt_full
vrt_full
<xarray.Dataset> Dimensions: (acq_date: 103, y: 396, x: 290) Coordinates: (12/17) * acq_date (acq_date) datetime64[ns] 2021-05-02T12... * x (x) float64 6.194e+05 ... 6.281e+05 * y (y) float64 3.102e+06 ... 3.09e+06 spatial_ref int64 0 sensor (acq_date) <U3 'S1A' 'S1A' ... 'S1A' 'S1A' beam_mode (acq_date) <U2 'IW' 'IW' ... 'IW' 'IW' ... ... masked (acq_date) <U1 'u' 'u' 'u' ... 'u' 'u' 'u' filtered (acq_date) <U1 'n' 'n' 'n' ... 'n' 'n' 'n' area (acq_date) <U1 'e' 'e' 'e' ... 'e' 'e' 'e' product_id (acq_date) <U4 '748F' '0D1E' ... 'BD36' granule_id (acq_date) <U67 'S1A_IW_SLC__1SDV_20210... orbital_dir (acq_date) <U4 'desc' 'asc' ... 'desc' Data variables: vv (acq_date, y, x) float32 dask.array<chunksize=(1, 396, 290), meta=np.ndarray> vh (acq_date, y, x) float32 dask.array<chunksize=(1, 396, 290), meta=np.ndarray> ls (acq_date, y, x) float64 dask.array<chunksize=(1, 396, 290), meta=np.ndarray>
vrt_full = vrt_full.where(vrt_full.vv != 0., np.nan, drop=False)
Read in vector data#
Manually-drawn outlines of proglacial lakes
lakes = gpd.read_file('https://github.com/e-marshall/sentinel1_rtc/raw/main/proglacial_lake_outline.geojson')
lakes_prj = lakes.to_crs('EPSG:32645')
lakes_prj
id | geometry | |
---|---|---|
0 | 1 | POLYGON ((623555.903 3099600.816, 623594.809 3... |
1 | 2 | POLYGON ((622405.254 3097521.050, 622268.598 3... |
Glacier outlines from Randolph Glacier Inventory
da_bbox = get_bbox_single(vrt_full)
rgi = gpd.read_file('https://github.com/e-marshall/itslive/raw/master/rgi15_southasiaeast.geojson')
rgi.head(3)
rgi_prj = rgi.to_crs('epsg:32645')
rgi_sub = gpd.sjoin(rgi_prj, da_bbox, how='inner')
rgi_sub.explore()
rgi_sub
RGIId | GLIMSId | BgnDate | EndDate | CenLon | CenLat | O1Region | O2Region | Area | Zmin | ... | Lmax | Status | Connect | Form | TermType | Surging | Linkages | Name | geometry | index_right | |
---|---|---|---|---|---|---|---|---|---|---|---|---|---|---|---|---|---|---|---|---|---|
2912 | RGI60-15.02913 | G088261E27938N | 20001108 | -9999999 | 88.260530 | 27.937820 | 15 | 2 | 0.222 | 5278 | ... | 667 | 0 | 0 | 0 | 0 | 9 | 9 | None | POLYGON ((624154.597 3091139.237, 624154.597 3... | 0 |
2913 | RGI60-15.02914 | G088296E27928N | 20001108 | -9999999 | 88.296067 | 27.928288 | 15 | 2 | 0.248 | 5372 | ... | 524 | 0 | 0 | 0 | 0 | 9 | 9 | None | POLYGON ((627398.396 3089890.401, 627403.687 3... | 0 |
2915 | RGI60-15.02916 | G088280E27949N | 20001108 | -9999999 | 88.279920 | 27.949292 | 15 | 2 | 0.166 | 5325 | ... | 589 | 0 | 0 | 0 | 0 | 9 | 9 | None | POLYGON ((626107.884 3092444.923, 626110.594 3... | 0 |
2916 | RGI60-15.02917 | G088289E27956N | 20001108 | -9999999 | 88.288797 | 27.955961 | 15 | 2 | 0.461 | 5351 | ... | 843 | 0 | 0 | 0 | 0 | 9 | 9 | None | POLYGON ((626663.114 3092564.217, 626654.813 3... | 0 |
2917 | RGI60-15.02918 | G088285E27952N | 20001108 | -9999999 | 88.284713 | 27.951895 | 15 | 2 | 0.169 | 5354 | ... | 682 | 0 | 0 | 0 | 0 | 9 | 9 | None | POLYGON ((626491.921 3092669.295, 626516.842 3... | 0 |
2918 | RGI60-15.02919 | G088277E27950N | 20001108 | -9999999 | 88.276515 | 27.950028 | 15 | 2 | 0.133 | 5403 | ... | 372 | 0 | 0 | 0 | 0 | 9 | 9 | None | POLYGON ((625706.190 3092218.712, 625675.749 3... | 0 |
2919 | RGI60-15.02920 | G088283E27949N | 20001108 | -9999999 | 88.282704 | 27.948794 | 15 | 2 | 0.052 | 5436 | ... | 456 | 0 | 0 | 0 | 0 | 9 | 9 | None | POLYGON ((626202.575 3092388.557, 626217.905 3... | 0 |
10460 | RGI60-15.10461 | G088226E27972N | 20100128 | -9999999 | 88.226000 | 27.972000 | 15 | 2 | 1.949 | 5410 | ... | 2435 | 0 | 0 | 0 | 0 | 9 | 9 | CN5O197B0032 | POLYGON ((620316.436 3095795.468, 620337.845 3... | 0 |
10461 | RGI60-15.10462 | G088239E27978N | 20100128 | -9999999 | 88.239000 | 27.978000 | 15 | 2 | 0.495 | 5654 | ... | 1807 | 0 | 0 | 0 | 0 | 9 | 9 | CN5O197B0031 | POLYGON ((621679.118 3094643.491, 621669.750 3... | 0 |
10462 | RGI60-15.10463 | G088251E27968N | 20100128 | -9999999 | 88.251000 | 27.968000 | 15 | 2 | 6.924 | 5335 | ... | 6668 | 0 | 0 | 0 | 0 | 9 | 9 | CN5O197B0030 | POLYGON ((624579.834 3093180.112, 624573.058 3... | 0 |
10463 | RGI60-15.10464 | G088271E27976N | 20100128 | -9999999 | 88.271000 | 27.976000 | 15 | 2 | 5.319 | 5328 | ... | 5609 | 0 | 0 | 0 | 0 | 9 | 9 | CN5O197B0029 Chutanjima Glacier | POLYGON ((626179.758 3095289.794, 626190.220 3... | 0 |
10464 | RGI60-15.10465 | G088276E28004N | 20100128 | -9999999 | 88.276000 | 28.004000 | 15 | 2 | 0.425 | 5758 | ... | 1266 | 0 | 0 | 0 | 0 | 9 | 9 | CN5O197B0025 | POLYGON ((625657.883 3097386.998, 625608.493 3... | 0 |
10465 | RGI60-15.10466 | G088277E28011N | 20100128 | -9999999 | 88.277000 | 28.011000 | 15 | 2 | 0.209 | 5808 | ... | 844 | 0 | 0 | 0 | 0 | 9 | 9 | CN5O197B0024 | POLYGON ((625439.972 3098924.917, 625433.875 3... | 0 |
10466 | RGI60-15.10467 | G088290E27983N | 20100128 | -9999999 | 88.290000 | 27.983000 | 15 | 2 | 3.744 | 5290 | ... | 5476 | 0 | 0 | 0 | 0 | 9 | 9 | CN5O197B0023 Mogunong Glacier | POLYGON ((626179.758 3095289.794, 626186.255 3... | 0 |
10467 | RGI60-15.10468 | G088299E27986N | 20100128 | -9999999 | 88.299000 | 27.986000 | 15 | 2 | 0.171 | 5623 | ... | 549 | 0 | 0 | 0 | 0 | 9 | 9 | CN5O197B0020 Yare Glacier | POLYGON ((627542.496 3096272.717, 627570.017 3... | 0 |
10468 | RGI60-15.10469 | G088302E28008N | 20100128 | -9999999 | 88.302000 | 28.008000 | 15 | 2 | 0.283 | 5477 | ... | 921 | 0 | 0 | 0 | 0 | 9 | 9 | CN5O197B0021 | POLYGON ((627882.258 3098849.454, 627882.729 3... | 0 |
10469 | RGI60-15.10470 | G088308E27981N | 20100128 | -9999999 | 88.308000 | 27.981000 | 15 | 2 | 3.625 | 5258 | ... | 3156 | 0 | 0 | 0 | 0 | 9 | 9 | CN5O197B0020 Yare Glacier | POLYGON ((629527.753 3096004.124, 629527.759 3... | 0 |
17 rows × 24 columns
rgi_sub = rgi_sub.loc[rgi_sub['RGIId'].isin(['RGI60-15.10463','RGI60-15.10464'])]
rgi_sub
RGIId | GLIMSId | BgnDate | EndDate | CenLon | CenLat | O1Region | O2Region | Area | Zmin | ... | Lmax | Status | Connect | Form | TermType | Surging | Linkages | Name | geometry | index_right | |
---|---|---|---|---|---|---|---|---|---|---|---|---|---|---|---|---|---|---|---|---|---|
10462 | RGI60-15.10463 | G088251E27968N | 20100128 | -9999999 | 88.251 | 27.968 | 15 | 2 | 6.924 | 5335 | ... | 6668 | 0 | 0 | 0 | 0 | 9 | 9 | CN5O197B0030 | POLYGON ((624579.834 3093180.112, 624573.058 3... | 0 |
10463 | RGI60-15.10464 | G088271E27976N | 20100128 | -9999999 | 88.271 | 27.976 | 15 | 2 | 5.319 | 5328 | ... | 5609 | 0 | 0 | 0 | 0 | 9 | 9 | CN5O197B0029 Chutanjima Glacier | POLYGON ((626179.758 3095289.794, 626190.220 3... | 0 |
2 rows × 24 columns
fig, ax = plt.subplots(figsize=(9,8))
power_to_db(vrt_full.vv.mean(dim=['acq_date'])).plot(ax=ax, cmap=plt.cm.Greys_r)
rgi_sub.plot(edgecolor='r', facecolor='none', ax=ax)
rgi_sub.plot(edgecolor='r', facecolor='none', ax=ax)
lakes_prj.plot(ax=ax, facecolor='none', edgecolor='blue')
fig.suptitle('ASF RTC backscatter image, 30 Apr 2022', fontsize=14);
Text(0.5, 0.98, 'ASF RTC backscatter image, 30 Apr 2022')
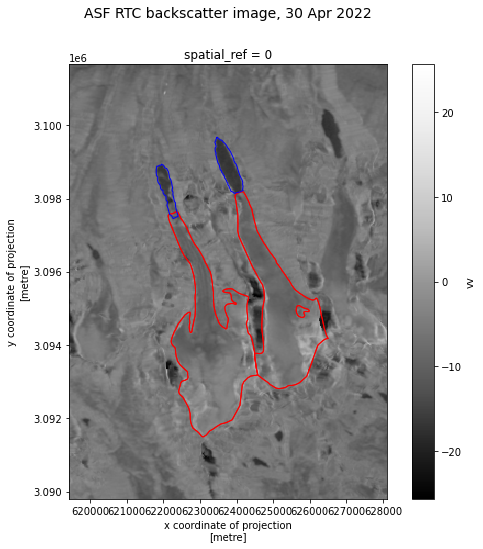
Clip to lake extent#
lake1 = lakes_prj.loc[lakes_prj['id']== 1]
lake2 = lakes_prj.loc[lakes_prj['id'] == 2]
lake1_asf = vrt_full.rio.clip(lake1.geometry, lake1.crs)
lake2_asf = vrt_full.rio.clip(lake2.geometry, lake2.crs)
lake1_asf
<xarray.Dataset> Dimensions: (acq_date: 103, x: 26, y: 51) Coordinates: (12/17) * acq_date (acq_date) datetime64[ns] 2021-05-02T12... * x (x) float64 6.234e+05 ... 6.242e+05 * y (y) float64 3.1e+06 3.1e+06 ... 3.098e+06 sensor (acq_date) <U3 'S1A' 'S1A' ... 'S1A' 'S1A' beam_mode (acq_date) <U2 'IW' 'IW' ... 'IW' 'IW' polarisation_type (acq_date) <U2 'DV' 'DV' ... 'DV' 'DV' ... ... filtered (acq_date) <U1 'n' 'n' 'n' ... 'n' 'n' 'n' area (acq_date) <U1 'e' 'e' 'e' ... 'e' 'e' 'e' product_id (acq_date) <U4 '748F' '0D1E' ... 'BD36' granule_id (acq_date) <U67 'S1A_IW_SLC__1SDV_20210... orbital_dir (acq_date) <U4 'desc' 'asc' ... 'desc' spatial_ref int64 0 Data variables: vv (acq_date, y, x) float32 dask.array<chunksize=(1, 51, 26), meta=np.ndarray> vh (acq_date, y, x) float32 dask.array<chunksize=(1, 51, 26), meta=np.ndarray> ls (acq_date, y, x) float64 dask.array<chunksize=(1, 51, 26), meta=np.ndarray>
lakes_prj['color'] = ['r','b']
lakes_prj
id | geometry | color | |
---|---|---|---|
0 | 1 | POLYGON ((623555.903 3099600.816, 623594.809 3... | r |
1 | 2 | POLYGON ((622405.254 3097521.050, 622268.598 3... | b |
Data visualization#
from matplotlib.lines import Line2D
fig, axs = plt.subplots(ncols = 2, figsize=(18,7))
#scatter plot VV
power_to_db(lake2_asf.vv.mean(dim=['x','y'])).plot(ax=axs[0], color = 'blue', marker='o', linewidth=0, alpha = 0.6);
power_to_db(lake1_asf.vv.mean(dim=['x','y'])).plot(ax=axs[0], color = 'red', marker = 'o', linewidth = 0, alpha = 0.6);
#scatter plot VH
power_to_db(lake2_asf.vh.mean(dim=['x','y'])).plot(ax=axs[1], color = 'blue', marker='o', linewidth=0, alpha = 0.6);
power_to_db(lake1_asf.vh.mean(dim=['x','y'])).plot(ax=axs[1], color = 'red', marker = 'o', linewidth = 0, alpha = 0.6);
axs[0].set_title('VV backscatter over proglacial lakes 2021-2022')
axs[1].set_title('VH backscatter over proglacial lakes 2021-2022')
legend_elements = [Line2D([0], [0], color = 'r', lw = 3, label = 'lake 1'),
Line2D([0], [0], color = 'b', lw = 3, label = 'lake 2')] ;
/home/emmamarshall/miniconda3/envs/sentinel/lib/python3.10/site-packages/dask/core.py:119: RuntimeWarning: divide by zero encountered in log10
return func(*(_execute_task(a, cache) for a in args))
/home/emmamarshall/miniconda3/envs/sentinel/lib/python3.10/site-packages/dask/core.py:119: RuntimeWarning: divide by zero encountered in log10
return func(*(_execute_task(a, cache) for a in args))
/home/emmamarshall/miniconda3/envs/sentinel/lib/python3.10/site-packages/dask/core.py:119: RuntimeWarning: divide by zero encountered in log10
return func(*(_execute_task(a, cache) for a in args))
/home/emmamarshall/miniconda3/envs/sentinel/lib/python3.10/site-packages/dask/core.py:119: RuntimeWarning: divide by zero encountered in log10
return func(*(_execute_task(a, cache) for a in args))
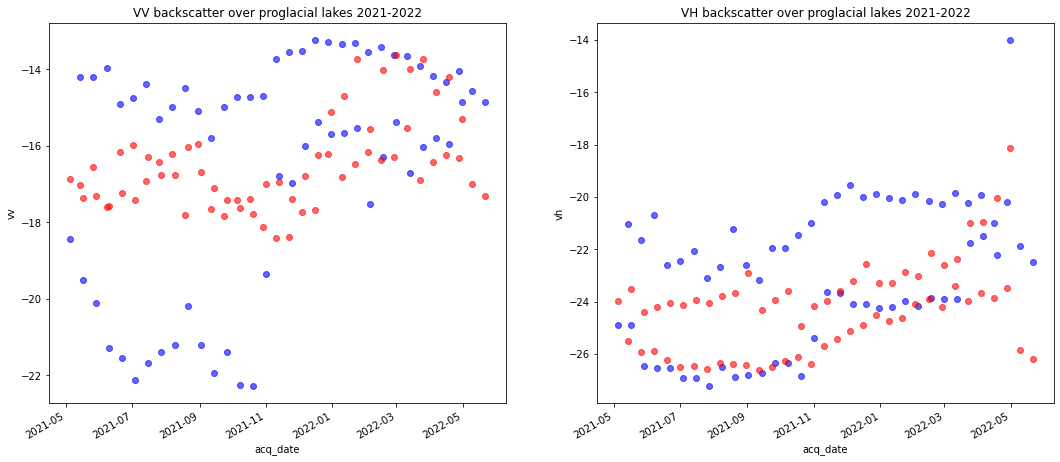
What observations can we make about VV and VH variability in the above plots? What would we want to look at next to further explore those observations?